Introduction
Every consumer, on numerous trips to retail stores, would have experienced decision fatigue while picking up a product, despite being clear on what is needed. During these moments, one always seeks the able assistance of a sales representative. With their deep knowledge and product experience, sales representatives are able to recommend the right product, thus being a critical cog in the overall shopping experience.
However, when it comes to the online shopping experience, there’s no digital version of the sales representative who can give each consumer a situational, contextual and personalized recommendation. This is where personalization engines come into play, as they are able to provide personalized recommendations about products that you can buy.
This article gives you an insight into everything that you need to know about personalization engines: what do they do, how they work and what capabilities t hey offer. This will help you decide if and how you can leverage it to meet your business goals.
The Use Cases
Scenario 1: Trying to find a specific product to buy
At Site A:
Let’s say you are shopping online at an ecommerce store at site A. You are looking for a black dress that has a a lace trim with floral patterns. While you have browsed b y category and searched for the terms black+dress+lace, you are, however, not able to find the product that meets your particular requirement.
If the same problem was encountered in a physical store, you would have reached out to the sales assistant who would have fetched products that meet your criteria based on your inputs. However, in an online store you have no such help available, where you are suggested products that may interest you.
You are frustrated after the long search and conclude that the s tore does not have a product that matches your requirement, and may even leave.
At Site B:
Consider you are shopping online at an ecommerce store at site A. You are looking for a black dress that has a a lace trim with floral patterns. While you have browsed b y category and searched for the terms black+dress+lace, you are, however, not able to find the product that meets your particular requirement.
As site B uses a product recommendation engine, at this instance, you are displayed a selection of products that are similar to the ones you have viewed on the site so far or meet the criteria of your search terms. Since this has greatly helped reduce your decision making time and enabled you to find the product you were looking for quickly, you, as a user, have been able to quickly complete your purchase and leave the site with good feedback, resolving to return again for more shopping.
You, as a user, are leisurely browsing the site with no decided intention to make a purchase. However, you are looking for the latest fashion, and if you find a good deal, you are open to making the purchase.
As site B uses a product recommendation engine, as you browse through a few products and visit the product details page, the Machine Learning (ML) algorithm of the product recommendation engine is able to learn from your actions and categorize your preferences to start suggesting similar products to you as you browse. This helps you to locate more such products that are in line with your taste in fashion, and you are pleased to find an item that is a steal. Thus, you complete
the purchase.
How do you help recommendation engines make suggestions?
Rule-based Personalized Recommendations:
When a segment of users is identifed due to a set of attributes they exhibit, rules are set up to determine what should be recommended to such visitors to engage them for a purchase.
ML-based recommendations with analytics reports:
ML allows a more data-centric approach where based on the past behavior of the user and current shopping preferences of similar users that led to sales, the algorithm suggests products to engage them for a purchase.
Trends in personalization
Meanwhile, we have seen a lot of advancements in the technology and adoption of personalization in terms of ML-based personalized recommenders:
- Advancement of ecommerce: Personalized recommenders are already adapted by various brand ecommerce sites.
- Customer expectations: Users have now come to expect recommenders to be a part of their shopping experience.
- User experience: People are already used to receiving recommendations and it has become an integral part of their life as they see them being offered by Netflix, Amazon, Spotify, Youtube etc.
- In fact, people are expecting recommendation engines to anticipate what they may need even before searching for it.
Moreover, an increase in revenue of about 5 to 15 per cent was evidenced by personalization leaders, as reported by McKinsey.
If you were to build your own personalization engines:
While we are already used to personalized recommendations such as ‘Products you might like’ on
eCommerce websites like Amazon, not all businesses that sell online offer the same kind of
experience. There are two ways a business may go about updating its site with product
recommenders for a personalized experience:
- Building your own ML-based personalization engines
- Use a recommendation engine built by third parties
Building your own ML-based personalization engines and validating their functionality for correctness is an avenue that may require investment in terms o f time, money and resources. If this is not for you, you may choose to opt for a SaaS model.
There are several mature recommendation engines available in the market which do the job; to name a few: IBM Watson Real-Time Personalization, Optimizely, Dynamic Yield, Rich Relevance, CubitPro, Evergage, Adobe Target, Monetate and HawkSearch.
How does ML power these product recommendations:
ML has become a common word in the technology lexicon. However, what problems does ML solve in the product recommender space? To be able to make effective recommendations that are specific to the particular consumer who is on your website at a particular time of the day, ML algorithms have to learn a few details about your products and services and what would be relevant to the user during this particular visit as opposed to his/ her other visits. Below are a few algorithms that were built to solve this problem:
- Content-based filtering method : Learns to classify the user's likes and dislikes based on an item's features. Refer Fig i below where as Jenna searches for a black dress, the recommendation engine identifies black heels that are tagged as a related item and sug gests it to her. Jenna is happy to purchase them as she realizes she does not have a pair of heels that would work with this outfit.
Fig i. Content based Filtering
- Collaborative-filtering method : Recommends based on reactions by similar users. Refer Fig ii below where the recommendation engine identifies Jenna and Anne as similar users based on their past purchase history and interests based on products visited on the site. Since a high percentage of users who meet this category of similar users have usually bought the black heels A when they purchased the outfit A (as denoted by Anne here), Jenna is also recommended the same high heels A for the outfit A by the recommendation engine.
Fig ii: Collaborative Filtering
- Hybrid method : Combination of content-based and collaboratove filtering methods
Other ML algorithms out there that try to address specific problems not addressed by the above algorithms include:
- Multi-criteria recommender systems: Uses users’ subjective preference ratings on multiple attributes of an item to recommend accordingly
- Risk-aware recommender systems: Takes into account the risk of disturbing the user with unwanted recommendations and recommends
- accordingly
- Mobile recommender systems: Uses internet access and smart phones to offer personalized, location-specific recommendations
Types of product recommenders
Based on whether the user input is consciously provided, the product recommendation solutions can be categorized in two types.
Explicit User Input-based: Works as a questionnaire which when answered, uses the inputs provided by the user to display a preset suitable solution. It is typically included in the signup process itself. For example, on websites like Netflix or Pinterest, the user is asked to provide their preferences in the sign up process after which relevant content is displayed. This is commonly used for digital products and services like movies, eBooks, articles, etc.
Fig iii Interests in categories explicitly shared by Lara during signup
Pros:
- Allows you to specify a set of products that are to be suggested based on the business rules that suit your industry
- You can define only a subset of products that need to be considered for a particular use case
- You can also suggest products that are low performers or on clearance that you need to sell
- User’s email ID can be captured at the end of a questionnaire. However, suitable legal text needs to be displayed in compliance with GDPR, CA Privacy Act
- Email IDs thus captured can be used for email targeting
- Serves both guest and registered users
- Serves as a value added experience to the user by being transparent as to why a certain product was recommended to them
Cons:
- If the user opts to not interact with the questionnaire, no dat a will be captured. This means that only a smaller subset of data is actually captured compared to the number of actual visitors to the site.
- If user chooses not to share the details, the recommender will not be able to share any results.
- Implicit user input-based: This recommendation engine studies any and all behavior (anywhere the user clicks) by the user based on interaction on the site to determine similar products that can be suggested. This is commonly used on brand ecommerce sites where users’ interactions with the site are used to predict what they are interested in so that recommendations are made accordingly.
Fig iv. Lara’s browsing behavior on the site captures specific interests
Pros:
- ML algorithms always suggest products based on the entire catalog, product attributes and user behaviors.
- Covers an entire catalog while suggesting the set of products to choose from
- It does not capture any Personally Identifiable Information (PII) data
- Serves both guest and registered users. However, the recommendations made will be more personalized for registered users based on their past interactions/behavior
- Data is captured for all visitors on the site who have JavaScript enabled in browser
- Report Data captured here can also be used to analyze the reasons for abandoned carts and other problems the website faces
Cons:
- If the user disables JavaScript in the browser, the required de tails may not be captured
- No specific way to identify products that are low performers to increase their sales by recommending them
- For new users, the recommendation will be more generic in nature
FAQs about product recommendation engines (implicit user input based) answered :
For any person looking to use recommendation engines to improve personalization on their website, one would have many questions to start with. Having used two such implicit user input-based recommendation engines, here are some answers to common questions.
What do they base their recommendations on?
Product recommender engines need two types of inputs to be able to make relevant recommendations:
- Master catalog data
- Implicitly acquired user behavior data
How do you implement it?
- Install/ integrate product with your site
- Send them your product feed/master catalog data
- Configure the recommendation strategy based on your use cases in their workbench
- Copy paste a snippet of code from their workbench that will automatically render product recommendations in your site
Does the integration need developer assistance? In our experience, yes.
- The development team may be needed to implement certain tags on specific pages through the consumer journey
- They may need to add some code to push user event data to tags
- Ensure that the recommender code copy pasted from the workbench is incorporated into your website pages if you do not have a what you see is what you get (WYSIWYG) editor to edit pages
Will this recommender carousel match the look and feel of my site?
Not exactly. However, they provide you the capability to change styles to match with your brand.
Will recommendations appear on any custom pages my site has?
Yes, this can be configured in the workbench as well.
I do not know what will work for my site. Are there any Out of the Box (OOB) recommendation strategies I can use?
Yes, such products provide you some generic recommendation strategies that would work for all domains or businesses. You can enable them and study the response they receive to enable custom or complex use cases in the future.
Typical OOB recommendation strategy use cases provided:
- Recently viewed by you, similar users
- Recently bought by you, similar users
- People also added to cart
- Top sellers
- Top rated
- Personalized recommendations (logic of how this is arrived upon is not exposed)
- Similar products
- Featured products
- Highly sought products
- Recently searched by you, similar users
What if our business desires customized recommendation strategies?
Such requests will have to be directed to the developer team of the product, which will help you customize the solution to meet your specific business needs.
What about new products included in my site?
To communicate to the recommendation engine about your new product, your scheduled product data feed will ensure that this data is communicated automatically. However, some of these recommendation engines do provide an option to receive a delta feed, which includes only the new product data to be fed to the recommendation engine. With this, the recommendation engine is updated about this recent change and these changes are reflected immediately on the live s ite.
To refresh the recommendation engine to accept this new data:
- Some of these products may enable you to refresh the recommendation engine, while for some others, this may reflect immediately once the delta feed is read .
To display the new product as a recommendation:
- As soon as the recommendation engine has data on this product, the latter will appear in any recommendation that meets the specific recommendation criteria.
How do I stop certain products from appearing as recommendations?
The workbench of these recommender engines allow you the option to blacklist specific products based on rules set up by you.
Do they store any PII data of the users that may not be compliant with privacy laws?
No user-specific data is stored.
In general, a specific user is only identified by a unique ID that is assigned to during the session, post which, actions on the site are monitored via clickstream data or cookies.
Do they suffer from cold start problem?
These products do not face the cold start problem as they have some inbuilt recommendation strategies which will continue to make suggestions based on order data captured for your site.
What product attributes are used to recommend the products?
The engine feeds on all the data available in the product feed shared to it. Some of these recommendation engines may identify key fields required as an input while others may read data from entire catalog. The details about whether certain product attributes are assigned a higher weightage than others is not exposed to us in the workbench.
Can a recommendation carousel only have one recommendation strategy feeding it?
There can be multiple recommendation strategies configured for a single carousel.
To illustrate, if your carousel displays four products at a time, two of the product recommendations can
be based on the Bestseller strategy and the other two on the Trending strategy. You can also set up fall
back strategies similarly.
For example:
You can mention fall back strategies in the below fashion:
Recommendation strategy Personalized has priority 1
Recommendation strategy Bestsellers has priority 2
Recommendation strategy Featured items has priority 3
What other controls are possible with respect to this carousel when it appears on my site?
- Here are some of the configurations possible with respect to the carousel:
- The number of products that should be displayed in the carousel
- Number of products that will be visible at a time in the carousel
- Enable/ disable autoscroll
- Specify the time duration for autoscroll
- Specify the time duration for which a certain recommendation strategy is to be active
- Priority of fall back strategies
- Number of fall back strategies
- Page-wise recommendation strategy set up
- Position on the page where the recommendations should appear
Product recommenders work well only for eCommerce or retailer sites, but would they work for brand/Direct to Consumer (DTC) sites?
Regardless of how expansive the number of products are and the variants featured on your site, every site desires to find ways to increase the visibility of its products to users to enable cross sell and upsell. The business may also desire to sell more of long tail items which is possible with product recommenders. The use of product recommendation engines by brands like Nike, Etsy etc. are proof of their effectiveness.
Product recommenders are known to work better than search as they help users find similar products that they may not be aware of. They are also known to reduce the product screening cost for consumers and enable quality decision-making.
Conclusion
To summarize, personalization engines are easy to integrate and are able to effectively highlight relevant products to the consumers at the right place and right time, which can result in increased sales. All this while providing a valueable service to the consumer by aiding the decision-making process.
Mindtree has been instrumental in implementing recommendation engines for various businesses and we have tried to share some of our learnings in this post. If you have any questions regarding the implemention of recommendation engines, do reach out to us.
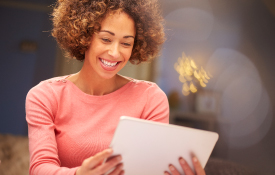