Client
An American multinational manufacturer and marketer of chemical products for consumers and industrial customers, the client operates more than 70 manufacturing, R&D, and operations facilities in over 30 countries and employs approximately 9,000 associates across four business divisions.
Challenge
The client had a standalone control system built on historical data to increase the throughput of an MDI plant, while maintaining the constraints within acceptable ranges. What the client wished to achieve was a streamlining the recommendation generation process for the control system so that the set point computation could be automated.
The two processes that required automation were the running of the constrained and unconstrained search, and the periodic denoising of the two year historical data. The client also wished to monitor the performance round the clock, and so required a centralized repository (data lake) on the cloud that would scale consumption by increasing the accessibility.
Solution
To begin the engagement with the client, Mindtree built a data lake that ingested data (ETL & transformation) from source systems including time series database (Aspen IP21). Then, we migrated the data wrangling process from R to Spark, thereby improving the performance & scalability. At the next step, we leveraged Databricks & Azure capabilities to scale the model to publish findings of 4 hour-slots using data that was refreshed every 15 minutes. After this, we rehashed, modularized, and built R packages for denoising and search to automate the model runs.
The solution build included an output visualisation that displayed the recommendations from the ML model.
Benefits
By providing advanced IOT analytics to scale supply chain processes, Mindtree delivered the following benefits:
- Automated the manual process of data ingestion & wrangling - what would earlier take 1-2 days now finished in a few minutes, based on source system data availability.
- Automated the recommendation generating engine for the control system. Client gets learns of changes in parameters 6 times in a day rather than once, thus enabling near real-time decision making that improves daily throughput and incrementally enhances yearly turn-over.
- Enabled the client to leverage the data team for elevated tasks
- Facilitated real-time reporting, which enabled on-time machine maintenance/handling, expanding asset life
- Enabled remote and near real-time accessibility to data with the central data lake, whereas earlier the client server architecture restricted remote access
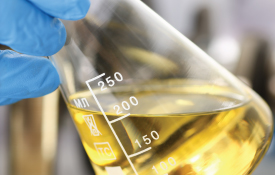