By Authors:
Subhasis Bandyopadhyay, BFS Practice Head, Mindtree
Sagar Sinha, Vice-President, Sales & Channels, APAC & Middle East, Tookitaki
As today’s world rapidly embraces digitization, the emphasis on fighting financial crime, money laundering, and terrorist financing using cutting-edge technology is on the rise. Due to the global phenomenon of digitalization, the amount of ‘wire’ activity is growing significantly, increasing the pressure on banks and financial institutions to monitor and detect suspicious activity prudently. This is a challenge as most banks still use archaic tools with an immature services ecosystem that depends on manual rules creation and lack self-learning capabilities.
Banks’ existing rule-based alert systems fall short of modern requirements as they address only a known set of scenarios. The result is a high percentage of false positives and negatives, while missing the truly suspicious incidents. This further leads to revenue loss, heavy penalties for non-compliance, and loss of brand reputation and image.
Challenges in modern financial crime prevention
Growing datasets, disparate transaction data systems, integration issues with monitoring systems, and changing regulatory norms are leading to new rule creation every time — a time-consuming and costly exercise. Hence, Artificial Intelligence (AI) and Machine Learning (ML) are coming into play in this space as banks need to move beyond a mere rules engine and must adopt dynamically adaptive predictive models or a dynamic workflow engine. This shift would enable real-time, transaction-based KYC anomaly detection, and AI/ML’s highly refined self-learning models focus on anti-money laundering detection and timely SAR (suspicious activity report) filing.
Recently, experts have realized the significance of analytics in combating money laundering. Most importantly, this pandemic resulted in the creation of shell companies to launder money as digital communication became a more common vehicle. Moreover, the pandemic has been used as an excuse to not meet the bank in person for compliance checks. This exposed banks to larger risks of financial crimes in client onboarding and relationship management.
Current Anti-Money Laundering (AML) compliance programs within banks are siloed with no or limited knowledge from peer banks. They lack a mechanism to share insights and patterns across banks, geographies, and even different business units within the same bank, resulting in insufficient and ineffective coverage of AML risks globally. While regulators are encouraging sharing of data and insights for better compliance, banks’ adoption rate on sharing and management of AML policies and dynamics is negligible.
Despite financial institutions and banks spending exorbitantly worldwide, there are several reasons why this investment is not having the impact that it should, including:
- Lack of transparency — Investigators often struggle to identify false positives quickly and accurately because money launderers have learned ways to bypass rules engines to avoid discovery.
- Lack of collaboration — Individual institutions are building their AML programs around their own specific products, customer types, and locations, with limited knowledge of suspicious patterns of behaviors being observed across their peers.
- Lack of resources — Financial institutions struggle to keep pace with implementing new rules. They are plagued with high false positive volumes and file too many low-quality or unnecessary reports because they are incentivized to cover their backs rather than applying sensible risk criteria.
In this context, regulators recognized that rules alone are not an effective method of detection and are thereby pressurizing banks to include more advanced analytics. The reason behind this is that financial institutions face challenges regarding:
- Setting up the correct threshold levels and parameters — When thresholds are set too low, the system will populate a high number of alerts that require analysis. On the other hand, if thresholds are set too high, the number of alerts will decrease, but the bank may not detect all suspicious activities and will fail to meet regulatory requirements, risking both reputation and exposure to fines.
- Identifying ‘false positives’ quickly and accurately — Analysis of alerts may be time-consuming. However, it must be completed with a sufficient level of scrutiny to ensure compliance with existing governance processes. False positives, which are likely to be the biggest challenge, should be identified at the right time and removed as quickly as possible.
- Complying with global and regional laws and regulations.
- Accurate and timely reporting.
- Streamlining operations to minimize costs.
Download Whitepaper to read more
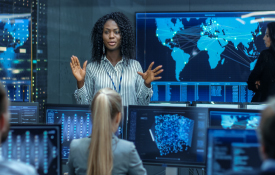